These days we rely on image sensors more than most people realize. They are in our vehicles, helping us avoid collisions, on buildings watching for intruders, and on production lines checking the quality of the goods we buy. Interestingly, they are often categorized by very simple metrics, such as pixel size or resolution, but choosing the best sensor for a specific application is much more complex.
Resolution
Image quality is crucial as we rely on sensors to detect hazards or find defects in manufactured products. System designers (and end users) often believe that higher resolution (more pixels in the image) leads to enhanced image quality. However, this is not always the case. Higher resolution will indeed retain sharper edges and finer detail in the image, which can aid object recognition—but there are additional considerations. A higher resolution impacts critical parameters, including capture speed/frame rate, sensor size, and sensor power. It also affects other system elements, as larger images require more bandwidth, storage, and processing power. Where higher resolution is necessary, reducing pixel size can maintain the lens and camera size to meet cost and size goals with enhanced image quality.
It is not uncommon for people to make assumptions that they need as many pixels as possible without considering the impact of their decision in terms of cost and system performance. At the start of a new project, a complete requirements analysis should begin with the end-use and the core parameters to meet that, with constraints such as physical size (for lens and camera body), power, or other limits. This approach will result in a sensor that matches your application needs better than if you restrict your selection by resolution too early in your evaluation.
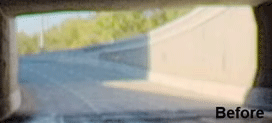
Figure 1: Resolution Before 1/1.5” 5.4 MP 3 µm Split-Diode Sensor
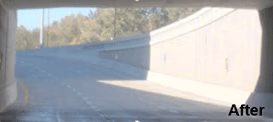
Figure 2: Resolution After 1/1.8” 8.3 Mp 2.1 µm Super-Exposure Sensor
Power Supply
Image sensor performance is also highly dependent on additional system components that may not be obvious because they aren’t in the optical path or even part of the sensor device itself. As a result, designers may compromise on aspects such as the power supply design. This approach will diminish image quality as electrical noise from the power supply components can cause image defects that can vary from subtle to something evident that every viewer would notice, even if they didn’t know the cause.
Essentially, image sensors are photon counters. In low-light conditions, the number of photons is low, so any “noise” in the system will be more noticeable in the image. Voltage spikes or voltage transients from the power supply can result in defects in the final image output. While sensors are designed for the power supply voltage to fluctuate within a tolerance range, any deviation outside that range may impact image quality. Therefore, the quality of the power feed is a crucial element of the camera system design.
Noise Sources
While it would be great to have a perfect device that measures light with no error or bias, in reality, the electronic circuits in a sensor die are subject to different noise sources that affect each pixel’s signal level and therefore affect pixels in the final image. Generally speaking, read noise is well-controlled with modern sensors, but another noise source, called Dark Signal Non-Uniformity (DSNU), is more challenging.
DSNU is what you would see for an image taken in complete darkness: it’s dark, so there should be no signal at all, but some electrons don’t behave perfectly, so they get counted as if caused by incoming light, and the picture will not be perfectly black. If this is the same for each pixel, it can be subtracted—just like you might edit a photo to make the whole image slightly darker. The problem arises when it is not uniform across the array, so DSNU is a measure of how much variation there is across the array, and it gets worse as the temperature of the sensor increases. Because it is affected by the temperature, a sensor might look good when tested in an air-conditioned laboratory but not if it is outside on a hot night. A hot, dark night is the most challenging for managing DSNU because there is not much valid signal; this noise source will be more visible. To address this, measure any sensor across the range of temperature and lighting conditions your system will see in regular use. If you select an image sensor based on room-temp tests, you might be surprised when temperatures rise.
Signal-to-Noise Ratio (SNR)
SNR, or signal-to-noise ratio, is defined as the average ratio of signal power to noise power. Regardless of how much noise you have, if you have a very high signal-to-noise ratio, then the impact of the noise on the image is much less noticeable. Think of this like an error on a restaurant check. If you only ordered a cup of coffee, a $3 extra charge would seem like a big deal, but if you had a large group of people and the bill is hundreds of dollars, you might not notice the extra charge because it is a small percentage error, even though it is $3 in both cases. Likewise, if you have a signal level from thousands of photons, you are unlikely to notice a few extras.
Coming back to image sensors, if you have an image that has bright areas and dark areas, you will observe more noise in some areas. Ironically, this may not be in the dark parts of the image, and it might be in the “mid-light.” Still, the design limits are exposed in transition areas where the method for low light transitions to the method for brighter light. This is challenging to explain without getting into deeper technical detail, but an analogy might be like gears on a bicycle. If you have a 10-speed bike, you’ll have a gear optimized for low speed, one for top speed, and lots of steps between those two. Now imagine you have only the top, a middle gear, and the bottom gear: you’ll have the right gear for going slow (low light), medium (mid-light), or fast (bright sunlight), but your transition from low speed to middle and middle to high will not be very comfortable, and you may find some parts of your journey really need one of this missing gears.
Some manufacturers often tout average SNR as a headline metric for image sensors, quoting performance statistics by cherry-picking areas where the SNR is good and implying that this represents the overall image quality across all lighting conditions. This is similar to the bicycle manufacturer quoting the average gear ratio for the 3-speed bike in our example. The middle gear is approximately the average of all 3, but the transitions from low to mid and mid to high leave a large gap where no selection is ideal. Designers must know this and look beyond the “average” SNR claims. The solution is to test the sensor for the range of lighting conditions needed for the application and to measure SNR across the entire range, trying to discover if you suffer from “missing gears” on your bicycle.
In short, if image quality is vital for your image sensor application, there are potential pitfalls you need to avoid. Assumptions about resolution and the impact of noise must be verified with testing to ensure you don’t have surprises in your final system design.